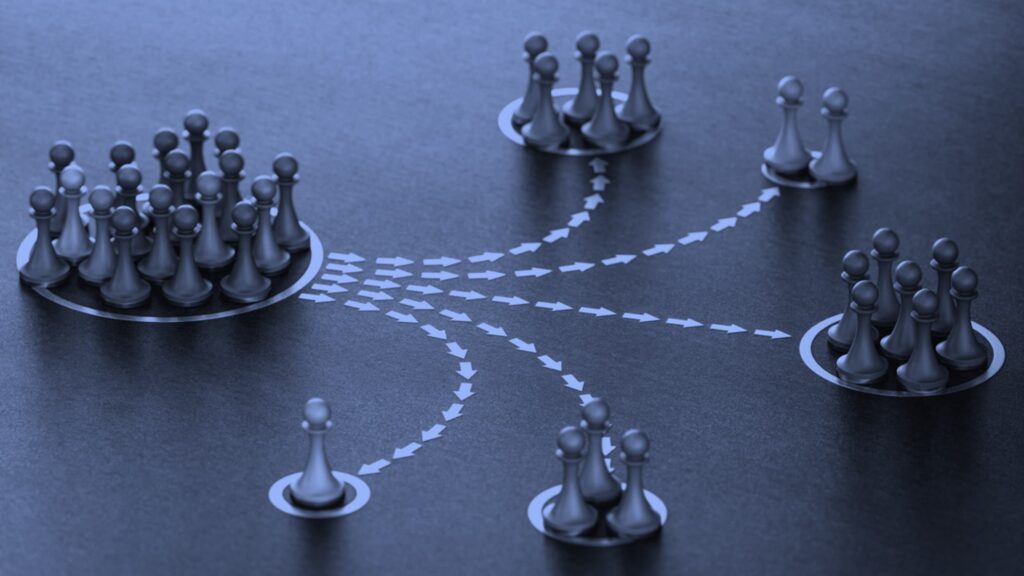
Removing hassles from segmentation and list building
We tried so hard to avoid the last-minute fire drills. Policies in place. Backup plans ready. Backups to the backups? Check! And yet, every few weeks…KABOOM! Colleagues would show up at my office door with an urgent communication request, their faces always red and their breathing heavy after the midday sprint from some other corner […]
02-07-23